Tuesday 4 June 2024
Part 2: Using AI in Redress and Remediation: Preparing for the Car Finance Mis-selling Regulations
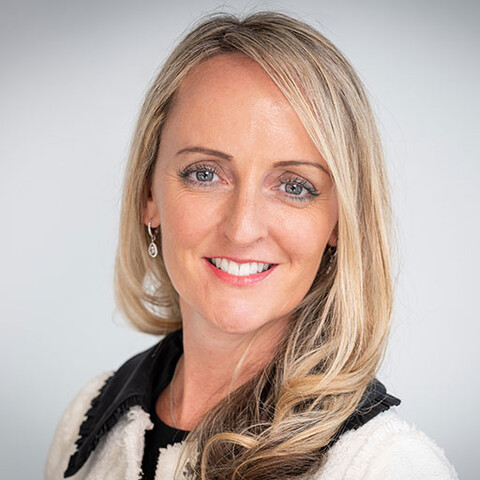
Charlene MCLAUGHLAN
Head of Sales and Marketing (Talan Data UK)
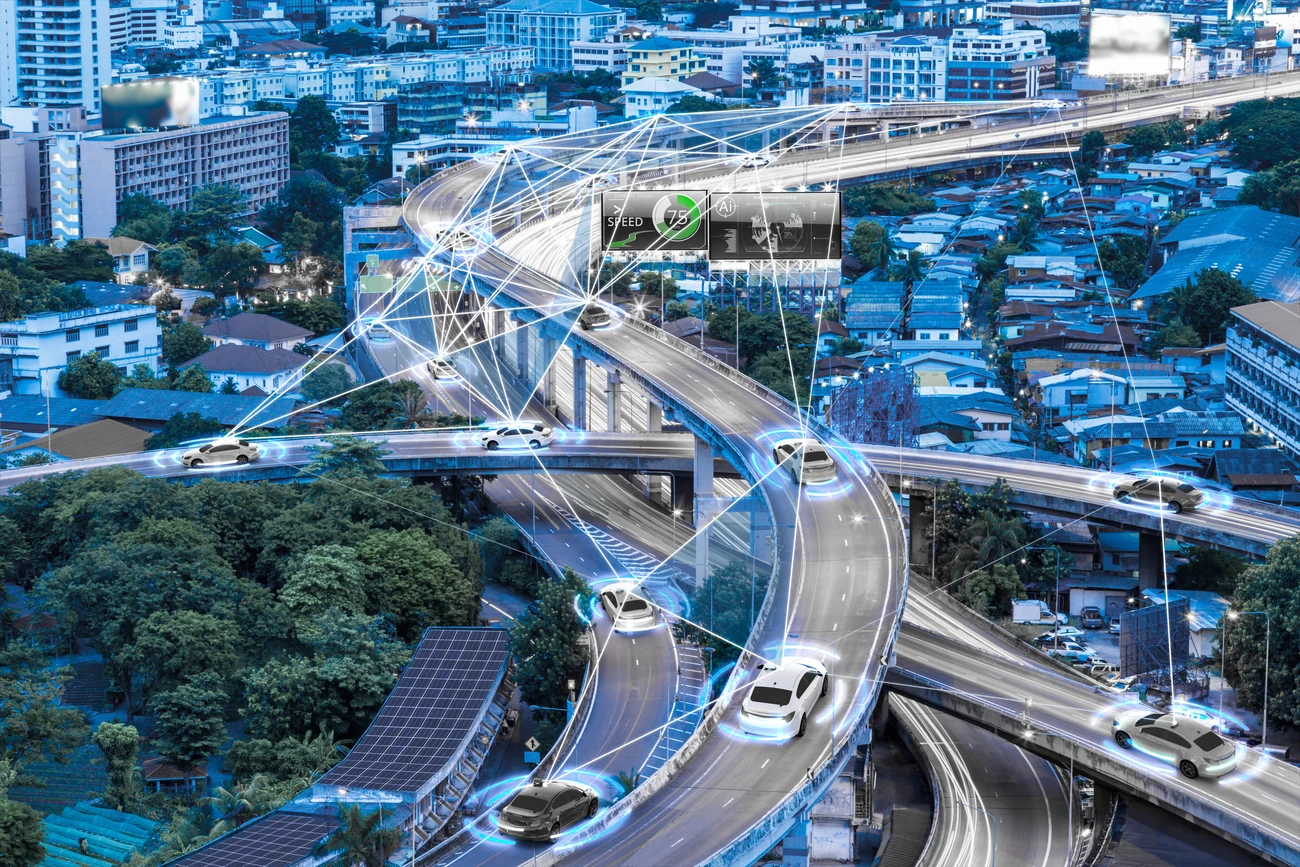
In this series, we are looking at how to plan ahead for potentially large-scale remediation projects, and how developments in technology, particularly Machine Learning (ML) and Artificial Intelligence (AI), can impact efficiency and cost-effectiveness in such projects.
Part 1 looked at the Discovery stage of a remediation project, encouraging our clients to begin preparation for September’s FCA ruling on the Discretionary Commission Agreements (DCAs). Whilst the results of the ruling cannot yet be known, there are always potential benefits and opportunities to be gained from taking steps to identify, cleanse and efficiently store customer data, whether current or historical.
We will now take a closer look at the elements of the Delivery stage of a remediation project, and where data/ML/AI driven solutions can help streamline various aspects. Talan UK’s 20+ years of experience with small, medium and large sized remediation projects has taught us that the following are key aspects of this part of the process:
- The effective holding, cleansing and improving of data, for example, to fix poor formatting of names and addresses
- The awareness of risks and identification of fraudulent claims and/or multiple claims on the same account
- The importance of effective communication and collaboration between departments and functions
Here’s where we would apply ML and AI to add efficiencies to the Delivery stage…
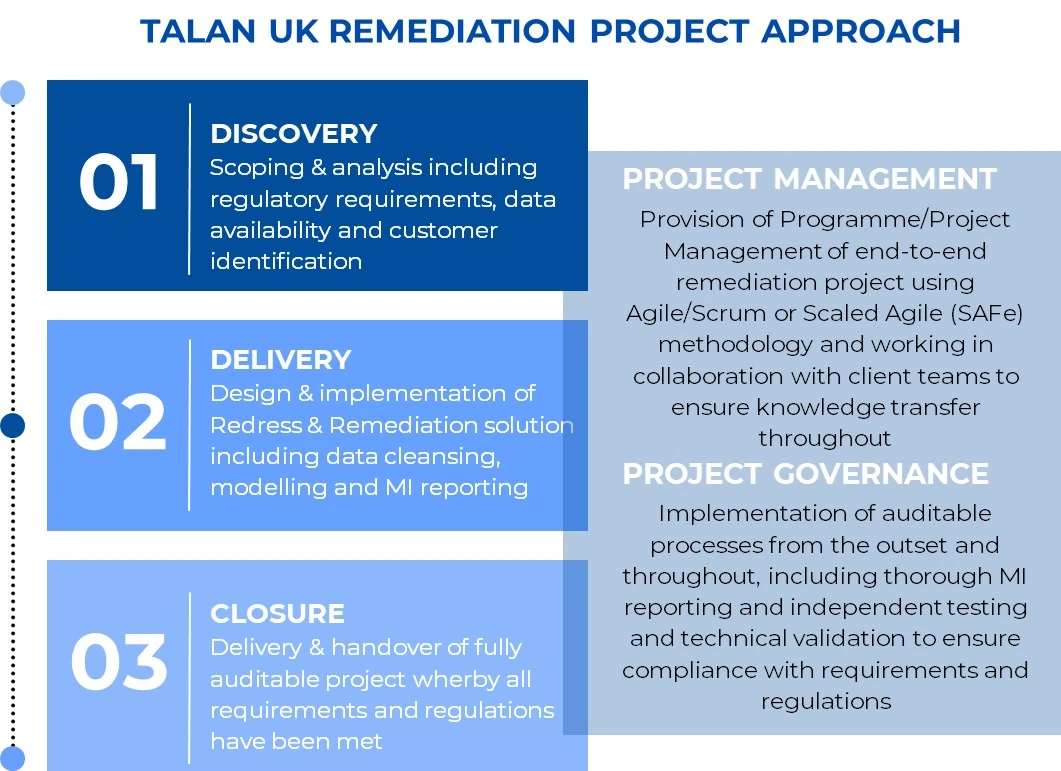
Redress Calculation Methodology
Utilising Python, algorithms can be developed to calculate redress amounts based on predefined methodologies. Techniques such as regression analysis and machine learning models can be introduced if uncertainty is present.
Redress Order Strategy
Predictive modelling, optimisation algorithms, and dynamic prioritisation techniques can be employed to determine the order of redress, considering factors like severity, demographics, and resource constraints. This could include the use of clustering to split redress into different groups which can then be handled in different sprints dependent on priority.
Redress Accounts
Automation can be employed for tasks like data entry and document generation. Natural Language Processing (NLP) techniques, such as summarisation and classification, aid in analysing customer communications for efficient handling of complaints and feedback.
Progress Tracking
Interactive dashboards, sentiment analysis, and anomaly detection techniques offer real-time insights into project progress. Python frameworks like Plotly Dash and scikit-learn facilitate the development of these reporting pipelines. Talan UK’s data scientists can work with front-end specialists to develop these.
Keep an eye out for Part 3 where will highlight which areas within the Closure phase of a Remediation Project are prime for efficiency gains through AI and ML.
For now, don’t hesitate to get in touch for more information on how Talan UK can help you get prepared AHEAD of September’s ruling.